내용추가: 24/10/10
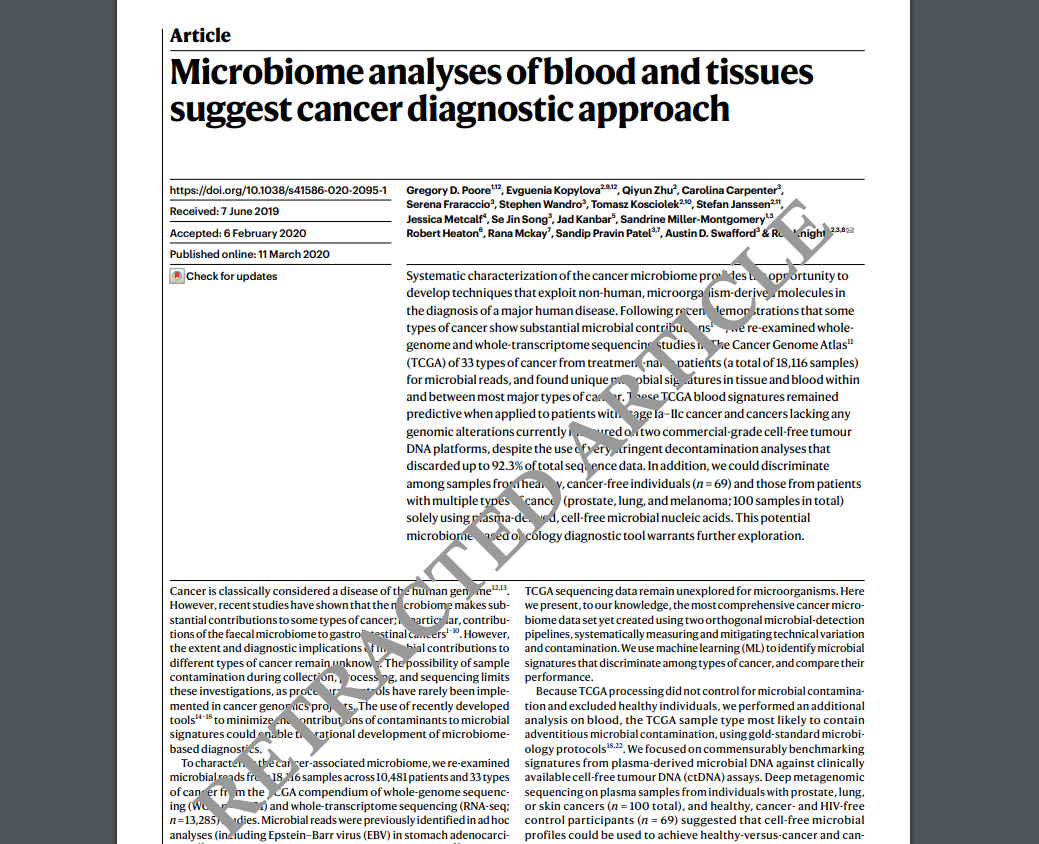
지난 6월, 네이처에 게재된 마이크로바이옴 관련 논문이 철회되었습니다.
해당 논문은 암 연구를 위해 활용된 WGS 데이터(TCGA)에서 박테리아 유전체를 추출하여 32가지 암을 구분하는 머신러닝 모델을 개발한 연구입니다. 이 연구는 Human Microbiome Project를 이끄셨던 Rob Knight 교수님의 연구실에서 수행되었습니다.
최근 레딧을 통해 이 소식을 접하게 되었습니다. 비록 이 주제가 매우 뜨거운 이슈임에도 불구하고 국내에서는 충분한 보도가 이루어지지 않았습니다. 이에 관련된 자세한 상황을 시간 순으로 정리하였으니 관심 있으신 분들은 참고하시기 바랍니다.
📅 Timeline
Cancer microbiome 저자 연구실은 🟣 , 반박의견은 🟢으로 표시
1️⃣ 2020년
Cancer mircobiome 논문에 네이처 등재 🟣
논문의 주장은 다음과 같다.
1. Non-human DNA 중 Genus에서 미생물로 판독된 결과를 가지고 암종을 구별할 수 있다.
2. 혈액 내 mbDNA로 초기 암을 구분할 수 있다.
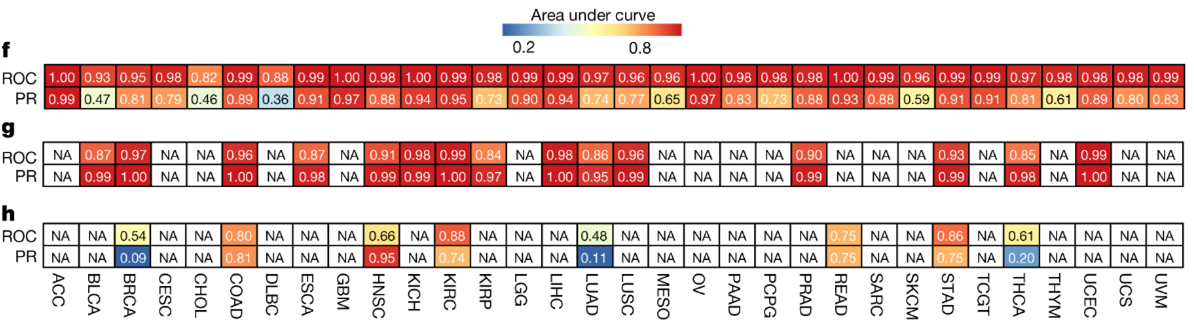
(Fig. 1f) 하나의 암 유형을 다른 암과 분류
(Fig. 1g) 종양과 정상조직을 분류
(Fig. 1h) 1기와 4기 암 사이 분류
모델의 정확도가 46~100%에 이르는 것을 볼 수 있다.
(Poore GD et al., Microbiome analyses of blood and tissues suggest cancer diagnostic approach, Nature, 2020)
2️⃣ 2022년도 9월
같은 실험실에서 진균으로도 암종을 구분할 수 있다는 연구 결과 발표 🟣
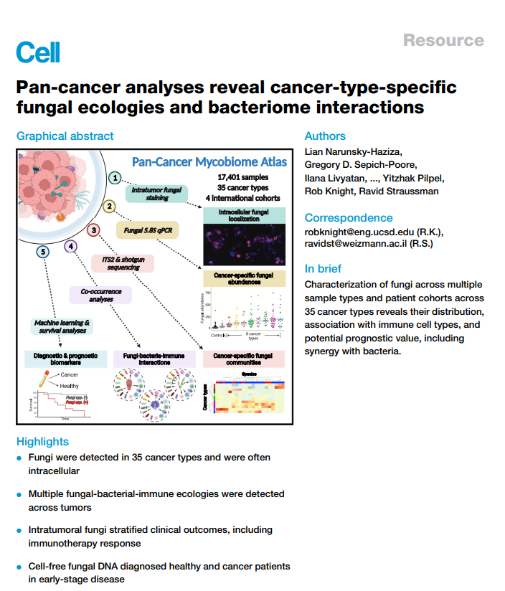
(Narunsky-Haziza L et al., Pan-cancer analyses reveal cancer-type-specific fungal ecologies and bacteriome interactions. Cell. 2022)
3️⃣ 2023년도 1월
Gihawi박사님의 위 논문에 대한 반박 제시 -> 현재 Mircrobial Genomics 게재 🟢
We believe there are issues in the areas of the contribution of contamination, handling of batch effects, false positive classifications and limitations in the machine learning approaches used
논문에 대한 반박은 다음과 같다.
1. 오염과 batch effect의 영향이 크다.
2. 기계학습 모델의 성능 판단 지표가 분명하지 않다.
3.ML 모델에서 비정상적인 Genus 가 중요하다고 판단되었다.
이에 대한 증거로, Poore의 논문에서 각 암 타입을 구분하는 데에 큰 importance score를 가진 미생물들이 사람의 몸이 아닌 극한환경에서 발견된 종임을 제시하였다.
Genus | Top feature in cancer type model | Details |
Leucothrix | Bladder cancer | Bacteria from marine macroalgae [ 5 ] |
Thalassomonas | Uveal melanoma | Bacteria causes disease in coral [ 4 ] |
Velarivirus | Cervical cancer | Grapevine is natural host [ 33 ] |
Tritimovirus | Colon cancer | Known to infect cereals [ 34 ] |
Dinovernavirus | Renal clear cell carcinoma | Contains insect viruses [ 35 ] |
Bacillarnavirus | Lung squamous cell carcinoma | Infects algae [ 36 ] |
Rymovirus | Ovarian serous | Infects species of grass [ 37 ] |
Ignicoccus | Prostate | Identified in marine hydrothermal vents [ 19 ] |
Salinimicrobium | Testicular cancer | Halophilic genus identified from marine environments [ 38 ] |
(Gihawi A et al., Caution regarding the specificities of pan-cancer microbial structure, Microb Genom, 2023)
4️⃣ 2023년도 2월
반박에 대한 답변 🟣
1. 오염과 batch effect의 영향이 크다
- WIS(Weizmann Institute of Science)에서 제공한 데이터로 분석을 수행
- Batch correction을 사용해도 결과는 유지되는 양상을 보임
"Moreover, a high feature importance score for a given taxon does not guarantee or imply an overabundance of that taxon for this comparison(Poore GD et al., Nature, 2020). "
(Gregory D et al., Reply to: Caution Regarding the Specificities of Pan-Cancer Microbial Structure, bioRxiv, 2023)
5️⃣ 2023년도 7월
추가 반박 논문 biorxiv 등록 -> 현재 mbio 게재 🟢
(i) errors in the genome database and the associated computational methods led to millions of false-positive findings of bacterial reads across all samples, largely because most of the sequences identified as bacteria were instead human; and
(ii) errors in the transformation of the raw data created an artificial signature, even for microbes with no reads detected, tagging each tumor type with a distinct signal that the machine-learning programs then used to create an apparently accurate classifier. ”
반박 내용은 아래와 같다.
1.Non-human DNA는 약 7% 가 아니라 0.5~2.5% 정도
- 기존 논문에서 사용된 Human genome은 GRCh37(hg19) 또는 GRCh38 임
- 최신버전인 CHM13 사용 시, unmapped read가 감소
- Poore의 논문은 구형의 Human genome을 사용하였고, Kraken의 database 또한 2016년도 버전임
2. 기존 논문의 결과에서 Genus의 count가 과장되어 있음
3. 정규화과정에서 생성된 데이터 오류
- Poore은 batch effect를 제거하기 위해 Voom-SNM 정규화 수행 (인용수 4,000)
- 모든 데이터를 0으로 변환 후, 동일한 과정 수행 -> 그럼에도 불구하고 암을 구별할 수 있음
- e.g. Hepandensovirus은 Raw data에서 read가 존재하지 않음에도, 정규화 이후 암을 구분하는 주요 신호로 사용됨
+) 동일한 실험 방법을 사용한 12개의 논문에 대해서도 결함이 있다는 것을 주장
(Gihawi A et al., Major data analysis errors invalidate cancer microbiome findings, mBio, 2023)
6️⃣ 2023년도 8월
논문 저자인 Poore의 반박 🟣
1. 암 종류에 따른 미생물 분포는 유의하게 다르다는 것은 여전히 입증 가능
- 전통적이고 보수적인 마이크로바이옴 분석 법(Shannon, Bray-Curtis)을 사용해도, 암에 따라 미생물 분포가 다르다는 것을 입증하며, 이는 97.59%의 정확도를 보임
2.WIS데이터와 Gihawi 데이터에서 중복으로 존재하는 Genus 만 사용
- 여전히 강력한 암 분류기 성능을 보임
3. 중복된 데이터에서 암의 종류를 잘 분류하는 9개의 종 관찰
- Pseudomonas, Staphylococcus, Clostridium, Acinetobacter, Streptococcus, Klebsiella, Prevotella, Fusobacterium, Veillonella 가 이에 속하며, 91,11%의 정확도를 보임
(https://github.com/gregpoore/tcga_rebuttal/tree/master)
Derek Lowe 논평가의 글 🟢
(https://www.science.org/content/blog-post/arguing-about-cancer-microbiome)
반박을 제기한 연구실의 교수님 트윗 🟢
- "주요한 반박(Batch effect, 정규화 방법의 문제)점에 대해 다루지 않았으므로, 아마 우리에게 동의하는 것이 아닐까?" by Steven Salzberg on X
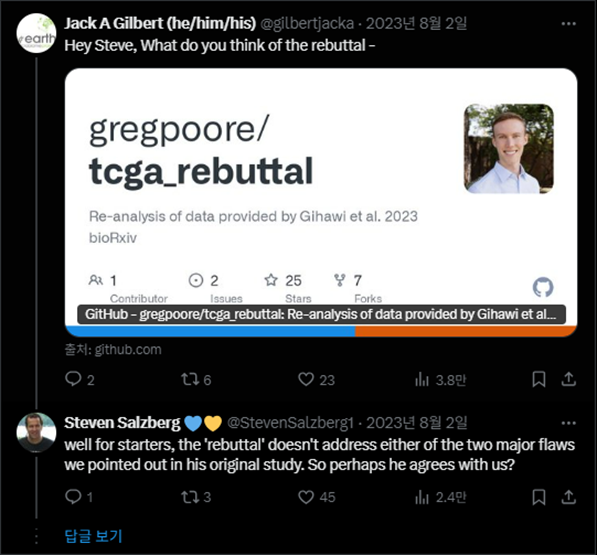
7️⃣ 2024년도 2월
Cancer microbiome 논문의 데이터가 잘 검증되었음을 알리는 반박논문 게재 🟣
(위 트윗을 보고 아마 반박 내용 위주로 논문을 작성하신 것으로 보임)
1.Batch effect에 관한 문제
- Voom-SNM으로 배치 교정된 데이터와 교정되지 않은 데이터 비교 => 기계 학습 모델 성능이 유사
- 또한, Batch effect를 감소시키는 최신 분석 도구 ConQuR 사용 시, Voom-SNM와 유사한 결과
2. 인간 유전체 오염 문제
- Conterminator, 가장 최신 Human Whole genome인 T2T-CHM13 사용
- 이는 데이터베이스문제가 아닌 Host gene 제거 방식의 차이
결론적으로, 수정된 결과에서도 원래 결론이 유효하게 나타남을 입증
(Sepich-Poore GD et al., Robustness of cancer microbiome signals over a broad range of methodological variation, Oncogene, 2024)
8️⃣ 2024년도 5월
Steven L. Salzberg 연구실 추가 논문 biorxiv 등록 🟢
기존에 반박을 위해 3개의 암 데이터만 사용한 것에 비해, 25가지의 암 데이터를 모두 분석한 결과를 공개.
정확한 리드부터 분석 코드 모두 공개하였음(물론 원 연구자도 분석 코드는 모두 공개하였음).
1.25가지의 암 종에서 Non-human gene은 극히 적음
2. 암과의 연관성
- 가장 풍부한 종: HPV~자궁경부암/ Bacteroides fragilis~직장선암(READ)
- H. pylori는 위선암(STAD)에서 17번째로 흔한 미생물
- 피부 미생물인 Cutibacterium acnes는 대부분의 암에서 풍부
3. 암의 종류와 연관성이 있다고 주장한 9종류의 속이, 재 분류 후 판독크기 감소
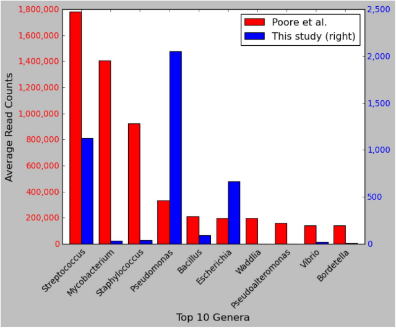
4. 진균 데이터베이스의 오염
- Narunsky-Haziza et al 에서 판독된 M.globosa는 재 분석 시 54,641 -> 4 read로 급감
- 이는 Genebank의 데이터 자체가 오염으로 판별됨 (GenBank accession GCF_000181695.1)
(Yuchen Ge et al., Comprehensive analysis of microbial content in whole-genome sequencing samples from The Cancer Genome Atlas project, bioRxiv, 2024)
9️⃣ 2024년도 6월
네이처 논문 철회
Retraction to: Nature https://doi.org/10.1038/s41586-020-2095-1 Published online 11 March 2020
The Editors have retracted this article. After publication, concerns about the robustness of specific microbial signatures reported as associated with cancer were brought to the attention of the Editors1. The authors have provided responses to the issues in a separate publication2.
Expert post-publication peer review of the issues raised and the authors’ responses has confirmed that some of the findings of the article are affected and the corresponding conclusions are no longer supported. All authors agree with this retraction.
- 철회: https://www.nature.com/articles/s41586-024-07656-x
- 관련기사: https://www.science.org/content/article/journal-retracts-influential-cancer-microbiome-paper
🔟 그 이후
해당 논문은 최종적으로 결국 철회되었으며, 철회된 논문을 인용한 다른 논문들도 주목받고 있습니다. Salzberg 교수님은 약 12개의 논문이 철회된 원 논문과 깊은 연관이 있음을 발표하였으며, 이에 대한 추가적인 결과를 지켜봐야 할 것입니다
이와 더불어 과적합 문제가 제기된 논문이 하나 더 있습니다. 과연 이 논문도 결국 철회될까요?
"Daria Beshnova et al., De novo prediction of cancer-associated T cell receptors for noninvasive cancer detection, Sci. Transl. Med., 2020"
연구 분야의 근본적인 문제
마이크로바이옴 연구의 가장 큰 단점은 현재의 기술이 실제 결과를 정확히 반영하지 못하고 있다는 점입니다.
일부 연구자는 "The microbiome is the bitcoin of biological research."라고 비판하였습니다. 이는 두 분야 모두 "아직 개발 단계이며 약간의 문제만 해결되면 완벽하다"는 평가를 받고 있기 때문이라고 합니다.
또한, 재현성을 고려하지 않고 전통적인 연구 방식을 소홀히 하며, 각 연구실마다 개별적인 분석 파이프라인을 사용한다는 점도 지적하였습니다.
연구자들은 각 도구의 자세한 사용법을 숙지하지 않고, 무분별하게 사용하는 것을 경계해야 한다고 합니다.
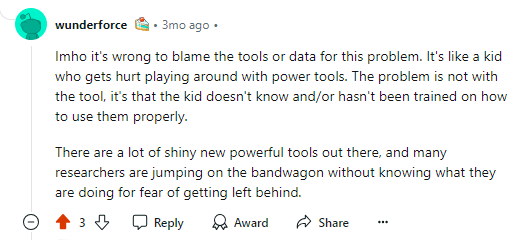
연구의 한계를 인지하고 이를 바탕으로 탐구하는 것이 중요하다는 생각을 하게 됩니다.
또한, 머신러닝(ML)은 유용한 도구일까요, 아니면 통계적 착각을 초래하는 걸까요? 확실한 것은 새로운 기술을 맹목적으로 신뢰하지 않는 것입니다.
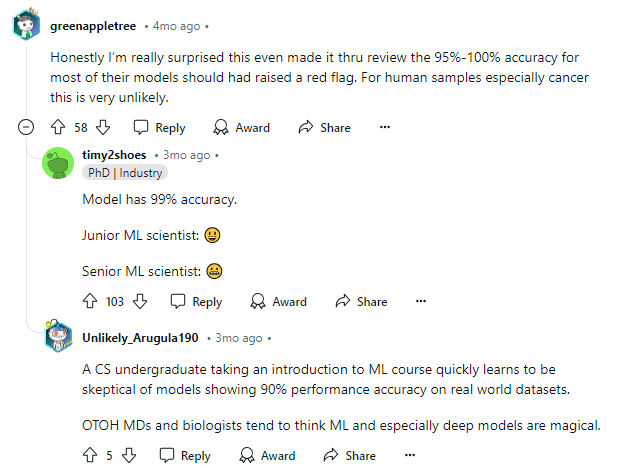
기타 참고글
- 관련 내용 정리 블로그: https://www.owlposting.com/p/a-primer-on-why-microbiome-research#%C2%A7tumor-microbiomes (추천👍)
이론! 반론! 오브젝션!
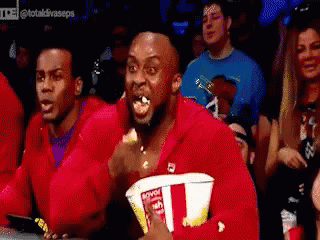
솔직히 모든 사건을 흥미롭게 지켜보았습니다.
Nature 저널에서 논문이 철회되는 사건은 매우 드문 사례이며, 그만큼 논문을 철회시킨 교수님들 또한 뛰어난 연구자들임을 인정합니다. 그러나 제가 존경하는 Knight 교수님이 부정적인 주목을 받고 있는 상황이 더욱 안타깝게 느껴집니다.
읽어주셔서 감사합니다.